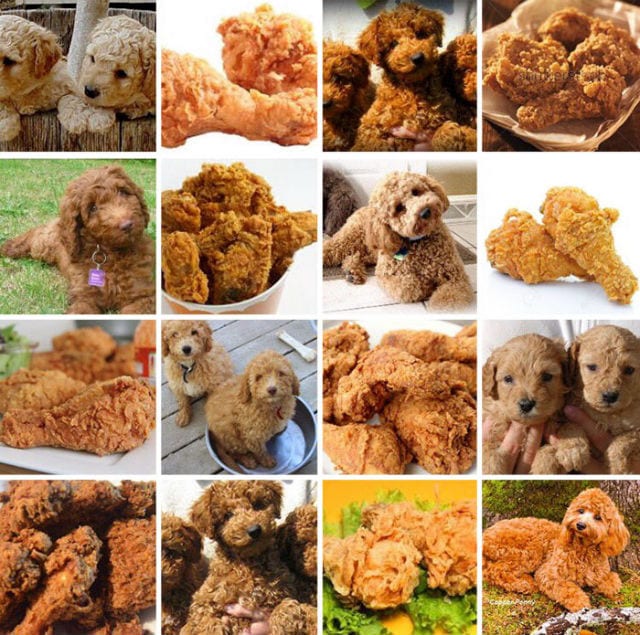
FPSim2 is the fast Python3 similarity search tool we are currently using in the ChEMBL interface. It's been a while since we first (and last) posted about it so we thought it deserved an update.
We've just released a new version (v0.2.0) and the highlights since we first talked about it are:
To give an idea of the scale and the timescale of the problem, we've calculated the matrix on chembl_27 (1941405 compounds) using 2048 bit, radius 2 Morgan fingerprints. 1941405 * 1941405 similarities is a lot of them! 3.7 trillion, exactly.
We've just released a new version (v0.2.0) and the highlights since we first talked about it are:
- CPU intensive functions moved from Cython to C++ with Pybind11. Now also using libpopcnt
- Improved speed, specially when dealing with some edge cases
- Conda builds avaiable for Windows, Mac and Linux. There is no Conda for ARM but it also compiles and works in a Raspberry Pi! (and probably will do with the new ARM Macs as well)
- Tversky search with a and b parameters (it previously had the 'substructure' feature with a and b respectively fixed to 1 and 0)
- Distance matrix calculation of the whole set feature is now available
- Zenodo DOI also available: 10.5281/zenodo.3902922
from FPSim2 import FPSim2Engine
fp_filename = 'chembl_27.h5'
fpe = FPSim2Engine(fp_filename)
csr_matrix = fpe.symmetric_distance_matrix(0.7, n_workers=1)
fp_filename = 'chembl_27.h5'
fpe = FPSim2Engine(fp_filename)
csr_matrix = fpe.symmetric_distance_matrix(0.7, n_workers=1)
To give an idea of the scale and the timescale of the problem, we've calculated the matrix on chembl_27 (1941405 compounds) using 2048 bit, radius 2 Morgan fingerprints. 1941405 * 1941405 similarities is a lot of them! 3.7 trillion, exactly.
Fortunately, the similarity matrix is symmetric (upper triangular and lower triangular matrices contain the same data) so we only needed to calculate (1941405 * 1941405 - 1941405) / 2 similarities. Still... this is 1.88 trillion similarities.
The role of the threshold is very important since it will help to skip a lot of calculations and save even more system memory. We can get the exact number of similarities that this calculation will need to do with a few lines of code:
1.2 trillion! The threshold saved 1/3 of the calculations and an insane amount of memory. But how much RAM did it save?
3.7 trillion vs 9.2 million results. Each result is made of 2 32bit integers and 1 32bit float. 44TB vs 110MB. Yes, terabytes...
The calculation took 12.5h in a modern laptop using a single core and 3.5h using 4.
The output is a SciPy CSR sparse matrix that can be used in some scikit-learn and scikit-learn-extra algorithms.
from FPSim2.io.chem import get_bounds_range
from tqdm import tqdm
sims = 0
for idx, query in tqdm(enumerate(fpe.fps), total=fpe.fps.shape[0]):
start, end = get_bounds_range(query, 0.7, 0, 0, fpe.popcnt_bins, "tanimoto")
next_idx = idx + 1
start = start if start > next_idx else next_idx
s = end - start
sims += s
print(sims)
1218544601003
from tqdm import tqdm
sims = 0
for idx, query in tqdm(enumerate(fpe.fps), total=fpe.fps.shape[0]):
start, end = get_bounds_range(query, 0.7, 0, 0, fpe.popcnt_bins, "tanimoto")
next_idx = idx + 1
start = start if start > next_idx else next_idx
s = end - start
sims += s
print(sims)
1218544601003
1.2 trillion! The threshold saved 1/3 of the calculations and an insane amount of memory. But how much RAM did it save?
print(csr_matrix.data.shape[0])
9223048
3.7 trillion vs 9.2 million results. Each result is made of 2 32bit integers and 1 32bit float. 44TB vs 110MB. Yes, terabytes...
The calculation took 12.5h in a modern laptop using a single core and 3.5h using 4.
The output is a SciPy CSR sparse matrix that can be used in some scikit-learn and scikit-learn-extra algorithms.
The order of the compounds is the same one than in the fps file (remember that compounds get sorted by number of fingerprint features). To get the fps ids:
ids = fpe.fps[:, 0]
Distance matrix can be easily transformed into a similarity matrix.
csr_matrix.data = 1 - csr_matrix.data
# 0's in the diagonal of the matrix are implicit so they are not affected by the instruction above
csr_matrix.setdiag(1)
# 0's in the diagonal of the matrix are implicit so they are not affected by the instruction above
csr_matrix.setdiag(1)
and also into a dense matrix as some algorithms, like MDS, require them:
# classic MDS doesn't work with missing values, so it's better to only use it with threshold 0.0
# in case you still want to run MDS on missing values matrices, this example uses the SMACOF algorithm which is known for being able to deal with missing data. Use it at your own risk!
from sklearn.manifold import MDS
dense_matrix = csr_matrix.todense()
# with metric=False it uses the SMACOF algorithm
mds = MDS(dissimilarity="precomputed", metric=False)
pos = mds.fit_transform(dense_matrix)
# in case you still want to run MDS on missing values matrices, this example uses the SMACOF algorithm which is known for being able to deal with missing data. Use it at your own risk!
from sklearn.manifold import MDS
dense_matrix = csr_matrix.todense()
# with metric=False it uses the SMACOF algorithm
mds = MDS(dissimilarity="precomputed", metric=False)
pos = mds.fit_transform(dense_matrix)
Bear in mind that, as shown before, to genenrate dense matrices from big datasets can be dangerous!
Comments